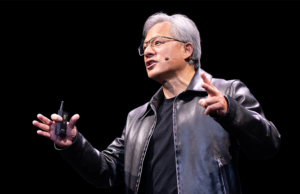
Jensen Huang at GTC
In a packed panel dialogue at GTC, moderated by NVIDIA Founder and CEO Jensen Huang, the architects of the groundbreaking transformer mannequin gathered to discover their creation’s potential. The panel featured seven of the eight authors of the seminal “Attention Is All You Need Paper” paper, which launched transformers — a sort of neural community designed to deal with sequential knowledge.
These highly effective neural networks deal with textual content and knowledge sequences in a manner that permits for quicker processing than older strategies. They do that utilizing a way referred to as “consideration,” which lets the mannequin concentrate on crucial data.
The transformer structure powers giant language fashions like GPT-4 and has ignited widespread curiosity in AI purposes throughout industries together with in biology, the place a lot knowledge might be represented in lengthy sequences. Transformers additionally play a supporting function within the not too long ago launched NVIDIA Inference Microservices (NIMs) and NVIDIA’s Blackwell GPU architecture, which relies on a second-generation transformer engine “purpose-built for accelerated computing and generative AI.”
Programming RNA molecules like organic techniques
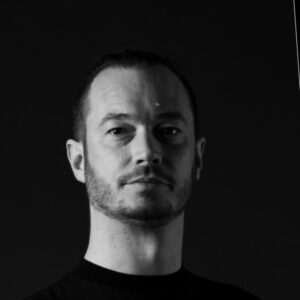
Jakob Uszkoreit
Within the panel, Jakob Uszkoreit, former senior employees software program engineer at Google and co-founder/CEO of the techbio startup Inceptive, shared his private motivation for exploring the potential of transformer fashions in biology. “In ’21, I cofounded Inceptive with the belief that there generally is a way more direct impression on bettering individuals’s lives with this expertise,” Uszkoreit stated. “My first baby was born throughout the pandemic, which gave me a newfound appreciation for the fragility of life.”
Inceptive has raised $120 million to date with most of it from a Collection A involving buyers like Nvidia and Andreessen Horowitz.
Uszkoreit’s curiosity in making use of transformers to molecular biology was additional piqued by two key developments. First, the success of AlphaFold 2 within the CASP14 protein structure prediction competition. AlphaFold 2 included transformer-based architectures, which contributed to its improved efficiency in comparison with its predecessor. “It turned actually clear that these things is prepared for primetime in molecular biology,” Uszkoreit stated.
Additional strengthening that thesis was the discharge of mRNA COVID vaccine efficacy outcomes. “It turned very clear that you are able to do something in life with RNA, however there was no knowledge for the longest time. In a sure sense, it was the uncared for stepchild of molecular biology,” Uszkoreit stated. “It simply appeared like nearly an ethical obligation. This has to occur.”
Uszkoreit envisions a future the place RNA molecules are programmed like organic techniques. “It begins as a program that you simply’ve compiled into one thing that would run on a GPU,” Uszkoreit stated. “In our case, the lifetime of a bit of organic software program begins with specifying the specified behaviors, akin to producing a selected protein in a cell at a sure degree. Then, we learn to translate that specification utilizing deep studying into RNA molecules that, as soon as in cells, exhibit these behaviors. The method goes past simply translating, say, English into laptop code; it additionally entails translating the specs of medicines and knowledge into precise molecules.”
Combining cutting-edge modeling with hands-on lab work
The method entails greater than computation. “It’s important to run experimentation towards nature,” Uszkoreit stated. “You actually should confirm this as a result of the info doesn’t but exist.” However there are “a ton of extraordinarily useful genomic knowledge that you could obtain, largely accessible brazenly and publicly as a result of it’s typically nonetheless largely publicly funded,” he continued. To handle this, Uszkoreit’s staff combines laptop modeling with hands-on lab work to generate knowledge “tailor-made to the particular phenomena you’re making an attempt to mannequin.” This is useful in areas like codon expression for mRNA vaccines, the place they’re breaking new floor. Their staff blends machine studying consultants and conventional biologists — a mix of robots and lab coats. “We consider ourselves as pioneers of one thing new,” Uszkoreit stated.
Transformers discovering use in drug discovery
Uszkoreit’s imaginative and prescient is only one instance of how the transformer structure is discovering use in drug discovery. A rising variety of different organizations are additionally experimenting with transformers to remodel drug discovery Exscientia, a UK-based AI drug discovery firm, is developing transformers to automate retrosynthesis and information the synthesis of recent drug molecules. Equally, Insilico Drugs’s Chemistry42 platform integrates transformers with different generative approaches to design novel compounds for drug targets.As well as, AstraZeneca skilled a model of its MolBART transformer mannequin on a big database of chemical compounds utilizing NVIDIA’s Megatron framework for coaching giant language fashions. The intention is for these fashions to check relationships between atoms in molecules, just like how language fashions be taught relationships between phrases, to help in drug discovery
Tutorial researchers, invoking the “Attention is all you need” tagline, are additionally exploring a wide range of transformer architectures to foretell drug-drug interactions, most cancers drug sensitivity and protein-ligand affinity.
Overcoming challenges of graph transformer fashions
Different efforts are aiming to discover strategies that overcome challenges related to graph transformer fashions. One key problem is distinguishing between isomorphic (structurally similar) graphs, which is essential for precisely representing and reasoning about molecular buildings. Demis Hassabis, the CEO and co-founder of DeepMind, alluded to this problem when introducing Isomorphic Labs, an autonomous subsidiary of Alphabet spun off from DeepMind. Isomorphic Labs focuses on AI-enabled drug discovery and goals to develop strategies that may seize the complicated nature of biology. “At its most basic degree, I believe biology might be considered an data processing system, albeit an awfully complicated and dynamic one,” Hassabis stated in a put up introducing Isomorphic. “Simply as arithmetic turned out to be the precise description language for physics, biology could develop into the proper sort of regime for the appliance of AI.”
“Biology is probably going far too complicated and messy to ever be encapsulated as a easy set of neat mathematical equations. However simply as arithmetic turned out to be the precise description language for physics, biology could develop into the proper sort of regime for the appliance of AI.”
The panelists additionally mentioned the way forward for reasoning in AI techniques. Llion Jones highlighted that the following frontier is to allow AI to cause extra powerfully by studying and looking for the precise architectures fairly than counting on hand-engineered approaches. “I believe the following massive factor that’s coming is reasoning, however I believe lots of people don’t notice this and lots of people are engaged on it,” Jones stated. He emphasised the necessity to discover the house of potential architectures and learn to wire them collectively to realize extra highly effective reasoning capabilities.
Filed Underneath: Drug Discovery, machine studying and AI
Tagged With: AI drug discovery platforms, AI in biotech, AI in Pharma, AlphaFold, computational biology, Drug design innovation, drug discovery, GTC, Jensen Huang, mRNA vaccine growth, NVIDIA, protein folding, protein construction prediction, RNA programming, Tranformers, transformer fashions, Transformer expertise